Ethics of Integrating Artificial Intelligence in Medicine
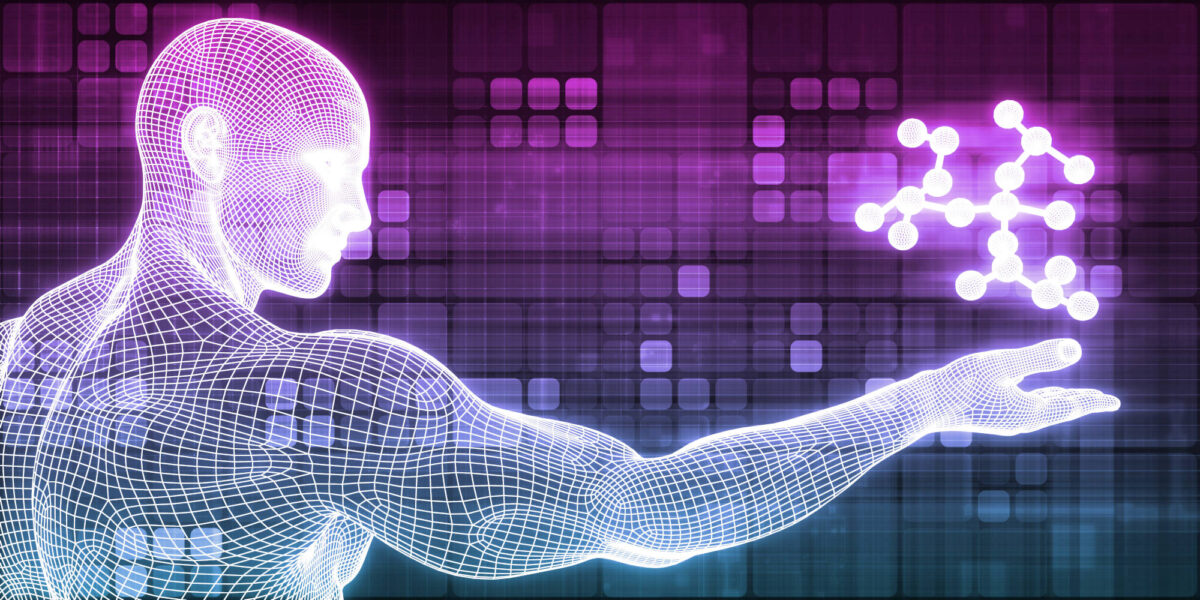
While the term “AI” or “artificial intelligence” has seamlessly woven itself into modern parlance, its precise definition remains vague. AI refers to an array of related but divergent technologies.1 Each promises its own contribution to the healthcare field, such as improving diagnoses, treatment plans, patient engagement, administrative services, and more.2 This range of applications creates a host of ethical quandaries, which some scholars have stratified across four fields: informed consent, safety and transparency, algorithmic fairness and biases, and data privacy.1 With over 40 AI-based medical devices already approved by the U.S. Food and Drug Administration and more artificial intelligence-based technologies supporting the infrastructure of healthcare systems, understanding the capabilities — and limitations — of these emerging technologies is critical to identifying and addressing the ethics of their integration into medicine. 1
The most widely utilized form of AI is “machine learning,” which is a process by which computational programs can improve their performances by accumulating data. Particularly complex forms of machine learning include “neural network” and “deep learning” models, which evaluate numerous variables and features to predict outcomes.2 For example, a computer program published by the Google Brain project is able to rapidly review imaging data to detect breast cancer with a reported sensitivity of 92.4%, which is significantly better than human sensitivity (73.2%).3 An article in the American Medical Association Journal of Ethics maintains that the decision not to use such a program could be unethical if, for example, a patient died as a result of a pathologist failing to identify a treatable cancer that a computer would have identified.4
Still, there are numerous ethical questions that arise in using such a program, which highlight matters of safety, transparency, and consent. One is the “black-box issue” — the question of whether it is important for patients and physicians to know how an AI program makes its decisions. The authors of the Journal of Ethics article emphasize that even if physicians are not entirely aware of how these highly accurate programs make decisions, the programs can augment pathologists’ work by helping them become more adept at identifying cancerous cells, thereby “illuminating” the black box. They emphasize the importance of the physicians’ technical expertise in using the AI, positing that the highest diagnostic accuracy could be achieved when human knowledge and skill is augmented by AI.4 Another Journal of Ethics article, describing the Watson artificial intelligence system developed by IBM and utilized in some medicine and health settings, recommends that hospitals require the programs to report “an audit trail with a minimum level of detail,” such as most heavily weighted variables, to help improve physicians’ practice and bolster patient-trust, empowering them to make decisions with informed consent.5
However, other ethical issues related to safety may still persist. For example, the increased reliance on AI could produce more “automation bias,” which occurs when physicians begin to defer to computer-generated results without considering their plausibility. Automation bias could result in missed diagnoses (false negatives) when machine-learning programs have failed to “learn” something a physician may be able to identify.4 Automation bias plagues even simpler forms of AI that have been implemented in healthcare. For example, “rule-based expert systems” are often imbedded in the infrastructure of electronic health record (EHR) systems—they use networks of “if-then” rules to support decision-making.2 They can aid physicians by alerting them of contraindications with planned procedures, or by sending precautionary notes for certain prescriptions. But these alerts can easily become clinical background noise: “Even if the system appropriately alerts doctors to every possible drug interaction, it won’t succeed if the doctors feel drowned by the blizzard of alerts and blindly okays them all just to get a prescription done,” Dr. Danielle Ofri writes in When We Do Harm. “Minor flaws in technology can cross-pollinate with minor human flaws, with the potential to multiply to a devastating end.”6
The interaction between human flaws and technological flaws is particularly important in considering questions of algorithmic fairness and bias.1 There are numerous instances of machine learning systems perpetuating existing biases through encoded modeling decisions or by detecting patterns in data that only represents certain populations. This is particularly likely to occur in the medical field because women and racial minority populations have historically been underrepresented in scientific research. For example, one paper examining a computer model found significantly different error rates in ICU mortality for race, gender, and insurance type and in 30-day psychiatric re-admission for insurance type. The authors conclude that a “closely cooperative relationship between clinicians and AI” could help undermine biases that may exist on the part of either actor.7 This level of algorithmic scrutiny highlights the need for physicians to have a high level of expertise in managing AI systems. But with the developers of such models largely working separately from the populations using them, strategies to promote better collaboration between these groups are urgently needed as more technologies become available.
As artificial intelligence finds more uses in medicine and other areas of society, it is critical for experts to continue examining the ethics of potential applications.
References
- Gerke S, Minssen T, Cohen G. Ethical and legal challenges of artificial intelligence-driven healthcare. In: Artificial Intelligence in Healthcare. Elsevier; 2020:295-336.
- Davenport T, Kalakota R. The potential for artificial intelligence in healthcare. Future Healthc J. 2019;6(2):94-98.
- Liu Y, Gadepalli K, Norouzi M, et al. Detecting cancer metastases on gigapixel pathology images. arXiv [csCV]. Published online 2017. http://arxiv.org/abs/1703.02442
- Anderson M, Anderson SL. How should AI be developed, validated, and implemented in patient care? AMA J Ethics. 2019;21(2):125-130.
- Luxton DD. Should Watson be consulted for a second opinion? AMA J Ethics. 2019;21(2):131-137.
- Chen IY, Szolovits P, Ghassemi M. Can AI help reduce disparities in general medical and mental health care? AMA J Ethics. 2019;21(2):167-179.
- Ofri, D. When We Do Harm: A Doctor Confronts Medical Error (Beacon Press, 2020).