EEG for Consciousness Assessment
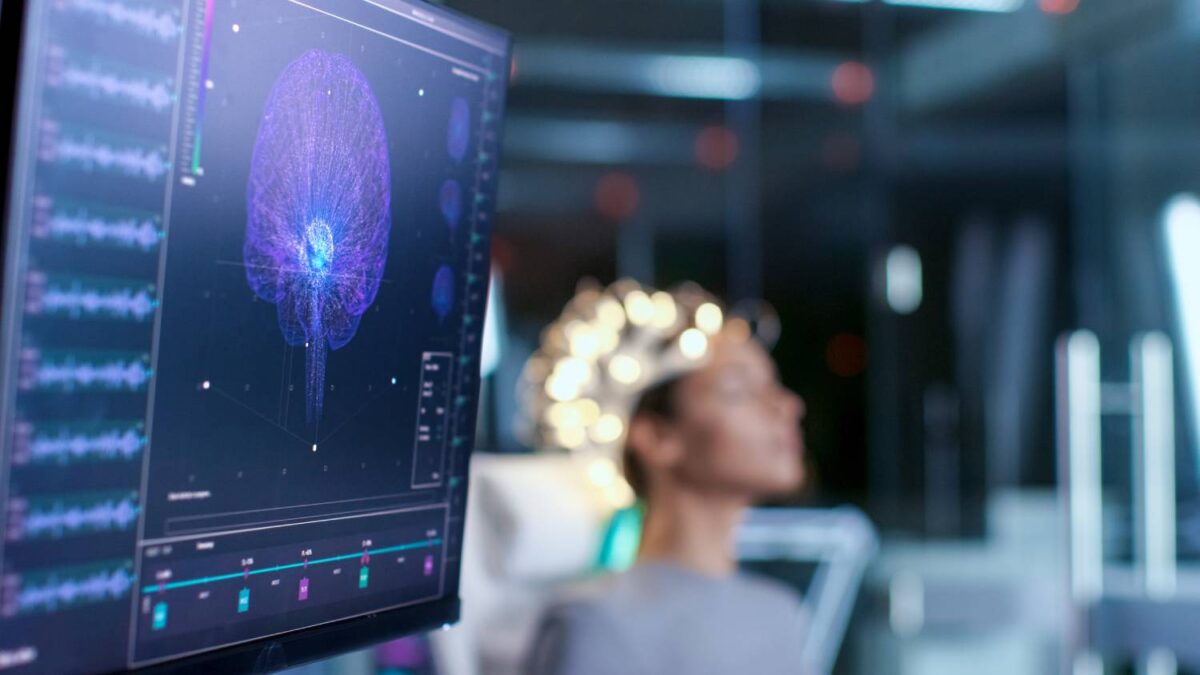
Probing consciousness remains a daily challenge for anesthesia providers. The electroencephalogram (EEG), invented in 1924 by German neurologist Hans Berger, is a dynamic, noninvasive, and relatively cost-efficient technique used to monitor the brain’s electrical activity. EEG recordings have a number of clinical uses that range from monitoring normal wakefulness or arousal states to complex clinical situations involving seizure or coma. Specifically, under general anesthesia, EEG recordings can be used to monitor the effects of anesthesia on the central nervous system and track patient consciousness.
EEG signals are acquired based on the brain activities of multiple brain regions over which arrays of electrodes are placed, generating many waveforms. The raw EEG is a complex signal with no clear, invariant signature across unconscious states; only limited information can be obtained from the EEG signals purely by waveform observation. As such, EEG recordings present many fundamental signal processing problems, including noise processing and high dimensional complexity. Advanced signal processing methods are necessary to automate signal analysis and translate the signal phenomena into meaningful, actionable markers (1,2). Pre-processing includes signal acquisition, artifact removal, signal averaging, output thresholding, signal enhancement, and edge detection. Next, distinctive or characteristic measurement, transform, or structural components are extracted from segments of EEG patterns in the process of feature extraction. Finally, signal classification is carried out by linear analytic, nonlinear analytic, or one of a number of alternative methods.
Linear signal transformation methods include a number of spectral analyses in the frequency, time, and time-frequency domains of the EEG recordings (3). In the frequency domain, these include the orthodox fast Fourier transform method, which analyzes the “frequency content” or distribution of signal power over a range of signal frequency values; in general, four core frequency bands contain the major characteristic waveforms of EEG spectra (delta, theta, alpha, and beta, in order of increasing frequency from 0.5 to 30 Hz). Resulting consciousness-associated patterns have long been well-established (4). In the time domain, methods include linear prediction, which estimates signal values based on a linear combination of past output values and past and present input values (5), as well as basic neurological data in some cases (6). In the time-frequency domain, techniques primarily include wavelet transformation, either continuous or discrete (7); in particular, depth of anesthesia is robustly reflected in phase coupling between delta and alpha sub-bands of EEG signals through continuous wavelet transformation analysis (8).
Arguably more accurately reflective of the way the brain actually functions, a number of nonlinear signal transformation methods have also been established (9). These take on the form of nonlinear parametric modeling, i.e. estimating the parameters of a model that will best fit the data in the time domain to build bottom-up generative models of whole-brain activity and states (10,11). In addition, dozens of different types of EEG entropy measures have also been developed, including response entropy and standard entropy, which assess the complexity or unpredictability of the EEG signal in frequency bands up to 32 Hz and 47 Hz, respectively (12). Normal waking states are associated with maximum values of entropy.
Finally, upwards of an estimated 255 EEG-based measures of consciousness in humans have also been established, taking on the form of adaptive algorithms, clustering techniques, and artificial neural networks (13) – each characterized by certain advantages and disadvantages.
Assessing the depth of anesthesia and disorders of consciousness remains a daily challenge for anesthesiologists. Specific methods should be selected and implemented based on context and goals. In the end, the applications of inferential analyses of EEG data to such diagnostic questions are broad and can help reduce errors in clinical care.
References
1. Abásolo D, Hornero R, Espino P, Álvarez D, Poza J. Entropy analysis of the EEG background activity in Alzheimer’s disease patients. Physiol Meas. 2006 Mar 1;27(3):241–53. doi:10.1088/0967-3334/27/3/003
2. Natarajan K, Acharya UR, Alias F, Tiboleng T, Puthusserypady SK. Nonlinear analysis of EEG signals at different mental states. Biomed Eng Online. 2004 Mar 16;3(1).
3. Al-Fahoum AS, Al-Fraihat AA. Methods of EEG Signal Features Extraction Using Linear Analysis in Frequency and Time-Frequency Domains. ISRN Neurosci. 2014 Feb 13;2014:1–7.
4. Nakata M, Mukawa J, Fromm GH. Evaluation of human consciousness level by means of “automated fluctuation analysis” of high frequency electroencephalogram fitted by double Lorentzians. Integr Physiol Behav Sci. 1993 Oct;28(4):343–52.
5. Stefan S, Schorr B, Lopez-Rolon A, Kolassa IT, Shock JP, Rosenfelder M, et al. Consciousness Indexing and Outcome Prediction with Resting-State EEG in Severe Disorders of Consciousness. Brain Topogr. 2018.
6. Claassen J, Velazquez A, Meyers E, Witsch J, Falo MC, Park S, et al. Bedside quantitative electroencephalography improves assessment of consciousness in comatose subarachnoid hemorrhage patients. Ann Neurol. 2016.
7. Adeli H, Zhou Z, Dadmehr N. Analysis of EEG records in an epileptic patient using wavelet transform. J Neurosci Methods. 2003 Feb 15;123(1):69–87.
8. Mousavi SM, Adamoʇlu A, Demiralp T, Shayesteh MG. A wavelet transform based method to determine depth of anesthesia to prevent awareness during general anesthesia. Comput Math Methods Med. 2014.
9. Stam CJ. Nonlinear dynamical analysis of EEG and MEG: Review of an emerging field. Vol. 116, Clinical Neurophysiology. Clin Neurophysiol; 2005. p. 2266–301.
10. Cofré R, Herzog R, Mediano PAM, Piccinini J, Rosas FE, Perl YS, et al. Whole-brain models to explore altered states of consciousness from the bottom up. Brain Sciences. 2020.
11. Bensaid S, Modolo J, Merlet I, Wendling F, Benquet P. COALIA: A Computational Model of Human EEG for Consciousness Research. Front Syst Neurosci. 2019.
12. Liang Z, Wang Y, Sun X, Li D, Voss LJ, Sleigh JW, et al. EEG entropy measures in anesthesia. Front Comput Neurosci. 2015 Feb 18;9(JAN):16.
13. Subasi A, Erçelebi E. Classification of EEG signals using neural network and logistic regression. Comput Methods Programs Biomed. 2005;78(2):87–99.